Keynotes
Quantifying Uncertainty in ASM Models with Markov Processes
Uwe Glässer, Simon Fraser University, Vancouver, CA
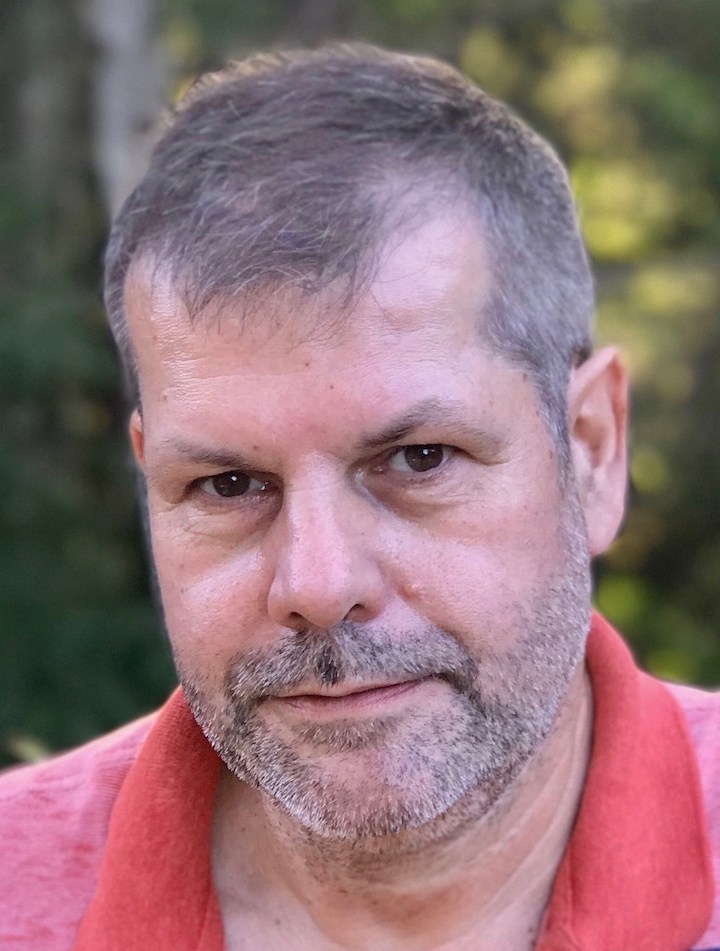
Describing discrete real world processes in the presence of uncertainty often allows for more realistic behavior predictions when combining qualitative modeling with quantitative aspects derived from observed data. Increasingly, historic data from past observations of real world process behavior is becoming available in amble quantities. We propose here a systematic approach to formally describe knowledge and understanding of discrete process behavior as abstract state machine models combined with probabilistic descriptions of behavioral patterns expressed in terms of Markov processes. In realistic scenarios, states of the underlying stochastic process are often assumed to be not directly observable, rather one can observe output signals associated with state transition events in a probabilistic sense. This view leads to hidden Markov models, allowing to quantify the likelihood of output sequences and related state transitions. We illustrate the approach for processes naturally characterized by time series analysis and forecasting in the context of situation analysis and anomaly detection.
RoboStar technology - a roboticist’s toolbox for combined proof and sound simulation
Ana Cavalcanti, University of York, York, UK
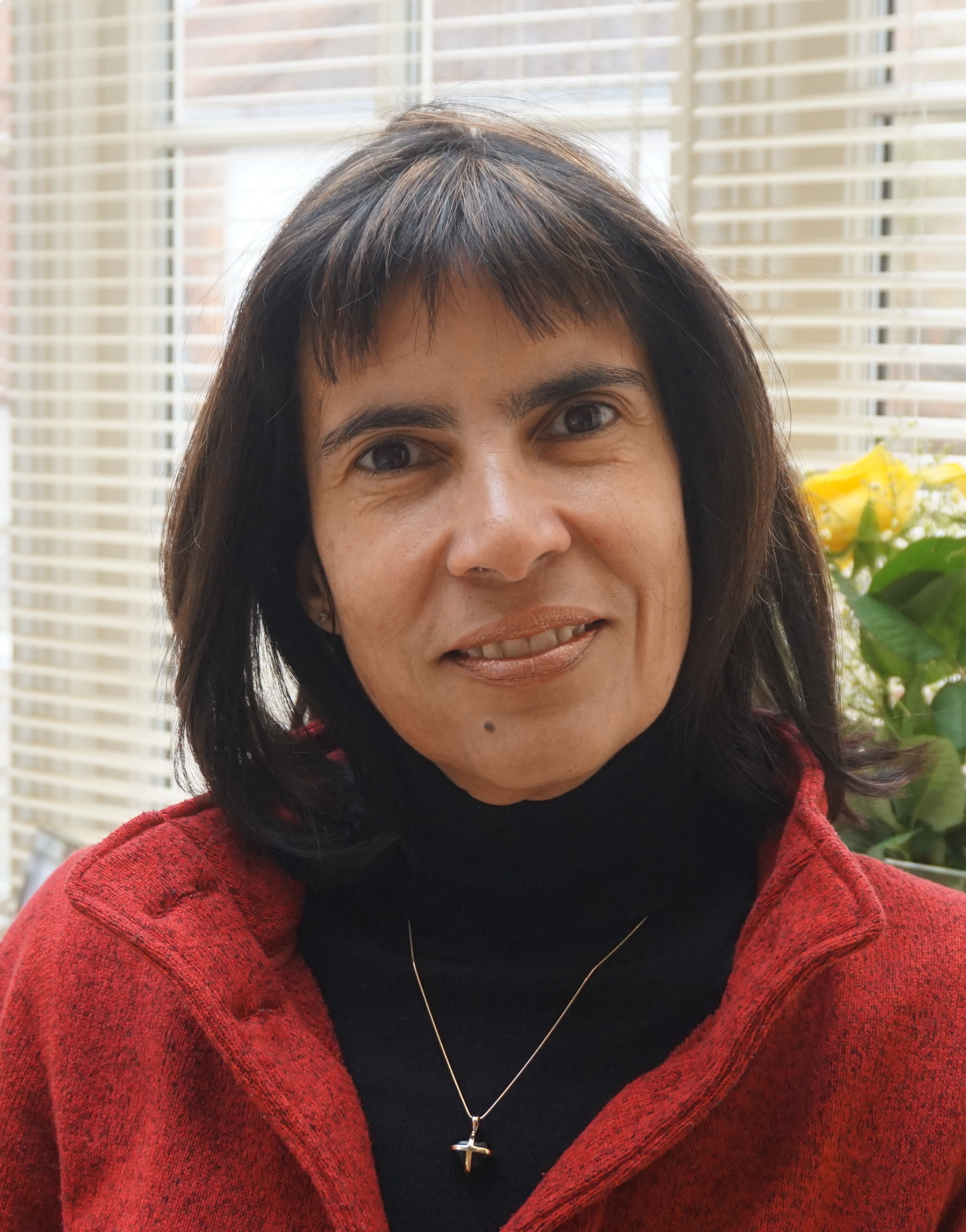
Simulation is a favoured technique adopted by roboticists to evaluate controller design and software. Often, state machines are drawn to convey overall ideas; they are used as a basis to program tool-specific simulations. The simulation code, written in general or proprietary programming languages, is, however, the only account of the robotic system. In this talk, we present a modern approach to design that supports the automatic generation of simulation code that is guaranteed to be correct, and complements the use of simulation with model checking and theorem proving.
This approach, under development by the RoboStar group (https://www.cs.york.ac.uk/robostar/), uses two domain-specific languages: RoboChart and RoboSim. RoboChart includes a controlled subset of UML-like state machines, a customised component-model, and primitives to specify timed and probabilistic properties. RoboChart is an event-based notation for design; RoboSim is a matching cycle-based diagrammatic notation for simulation. RoboSim also includes block diagrams enriched to specify physical and dynamic behaviours of robotic platforms.
Both RoboChart and RoboSim can be used to generate automatically mathematical models that can be used for verification. In the RoboStar approach, the mathematical models are hidden from practitioners, but can be used to prove properties of models, and consistency between designs and simulations. We have experience with FDR, PRISM, and Isabelle.
RoboChart and RoboSim can complement approaches that cater for a global view of the system architecture by supporting modelling and verification of the functional component-behaviour, covering interaction, time, and probabilistic properties. It also complements work on deployment of verified code.
Gilles Dowek, INRIA/ENS Paris-Saclay, France
Will be continued